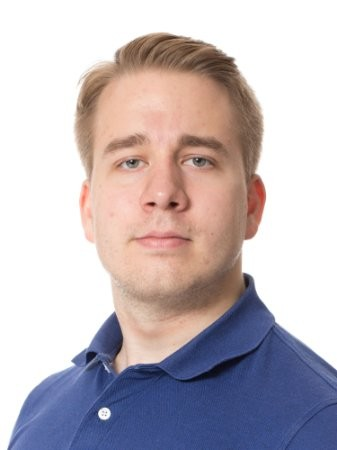
Hello! I'm Antoine. Welcome to my website. I am computer programmer and electronics enthusiast from Finland. I work at Qvantel as a software architect for the telecom sector. Before that, I used to work in logistics optimization, before that I did research at a university, and way before that I got my start in web design.
On this site you can find various content related to computers, electronics and all sorts of fun nonsense. There’s a blog, in which I post rather infrequently.
If you have any comments or suggestions you can discuss at my public inbox which is a mailing list serving as a discussion forum for my projects, including this website. Preferably, use the mailing list unless your message for one reason or the other is private, in which case you can use my personal mail address. You can send me patches both on the mailing list or my personal address.
Recent changes on July 28, 2021.
Site contents
- Programming, Lisp, software development practices
- Software I've written, libraries, games
- Emacs, the extensible, self-documenting text editor
- This site, about the site, its origins, its design rationale, or how it works
Latest blog posts
-
December 08, 2020Guile fun times, and blog update
-
October 11, 2020Divergence: A website is born
-
October 06, 2020No more microblog
-
October 05, 2020Between two Lisps
-
September 09, 2020Back to Rmail
-
September 03, 2020Inside the machine
-
March 07, 2020Winding down
-
November 20, 2019Embedded Rust and WiFi
-
March 09, 2019Configurability
-
September 16, 2018The runtime configuration problem